Marie-Luc Arpin, professor at the School of Management (Université de Sherbrooke); Sébastien Gambs, director of the Canada Research Chair in Privacy and Ethical Analysis of Massive Data and professor at the Department of Computer Science (UQAM)
Introduction
Over the past decade, the meaning of the word “urbanization” has undergone a surprising and structuring change. Today, against the backdrop of crises (environmental and health, social and humanitarian) which urbanization has become associated with (UN, 1976, 2016), the word evokes above all a set of problematic phenomena, if not a crisis in itself. Concurrently, however, urbanization is becoming a new vector of hope: “a transformative force” from which “sustainable and equitable urban futures” can emerge (UN-Habitat, 2016). In the fight against climate change, in particular, cities are now assuming a decisive role in planning and operationalizing the transition of behaviours toward “low-carbon” lifestyles (IPCC, 2022; Revi et al., 2014).
Still, confidence in this transition is not a given. It brings blind spots and comes with implicit promises and conditionalities regarding digital technologies which, rightly and wrongly, are becoming more prevalent as the climate crisis deepens (IPCC, 2022; Palm and Nikoleris, 2021). Massive flows of data, particularly from mobile devices for individual use, are significantly (re)shaping urban dynamics, so much so that any urban leadership in climate action will have to integrate it into its vision. While megadata are giving citizens and communities new capacities for adaptation, they are also making them vulnerable in new ways. In this sense, the opportunities brought by contemporary urbanization are a double-edged sword, and urban climate action can no longer be conceived in isolation from the (paradoxical) social dynamics of digital technology.
1) Literature review: Digital technology as a vector for urban climate action
In this article, we summarize the findings of the research report Synthèse des états de l’art sur les enjeux éthiques de la ville intelligente (Synthesis of the state of the art of the ethical challenges facing the smart city), authored by us and published in 2022. Our focus lies on megadata (big data) and the artificial intelligence (AI) systems that enable their analysis. We also discuss the “smart city,” which refers to the vast and largely imperceptible influence of AI in the urban context, particularly when deployed by platform companies (big tech). Apart from this dominant trend, other types of use of digital technology are possible. This focus is warranted because the double paradox which characterizes AI illustrates clearly the social dynamics that, if more subtly, accompany other technologies (5G, the Internet of Things, blockchain, etc.) and their integration in the urban context.
As posited in the research report, which draws on a corpus of literature with titles or abstracts that included the key words “city,” “digital,” “ethics” or their synonyms, the social science literature often fails to recognize this double paradox insofar as it frames and highlights the challenges of digital technology in urban environments as a problem, in other words, it subjects digital issues in urban environments to the soluble-problem model1 (Vérin, 1984). That said, the social science literature does effectively document the challenges of digital technology in cities. In this sense, it can serve as an empirical basis for theorizing the relationship between urban climate action and digital technologies. The literature demonstrates that in the second half of the 2000s the previously pluralistic discourse of the “smart city” became the preserve of large specialist companies2 (Albino et al., 2015; Halpern et al., 2013, 2017; Hollands, 2008). Through these companies, data collection, processing and management practices in urban environments were developed with disregard for (or exploitation of) the imbalance of forces and knowledge. These relationships have been poorly framed to date and are subject to various forms of abuse that need to be denounced (CEST, 2017, 2020; Hatuka et al., 2020).
From the outset, these companies based their rhetoric on environmental urgency and the supposed need to generate new knowledge for combatting it (Halpern et al. 2017). This rhetoric was essentially retained as the discourse transitioned to the so-called science of the city (Caprotti et al., 2017; UN-Habitat, 2016), which, fueled by the megadata that are constantly circulating within it, seeks to optimize mobility and energy efficiency, agriculture, crisis management and so on. However, this highly mediatized discourse fails to sum up the relationship between digital technology and urban climate action. On the contrary, since “everyday life is invented with a thousand ways of poaching” (de Certeau, 1990, p. xxxvii; own translation), other trajectories exist, as the literature reports. The AI solutions promoted under the dominant rhetoric so far tend to exacerbate the historical issues of urbanization—for example, in terms of socioeconomic disparities, exclusion of marginalized populations or environmental injustice (Bender et al., 2021; Green, 2019; Hatuka et al., 2020)—but urban climate action nonetheless remains driven by spontaneous adaptation strategies, new collaborative modalities or unusual alliances made possible by participation in megadata flows and citizen appropriation of AI (Guldi, 2016, 2018; Picon, 2015, 2016). The uses of digital technology go far beyond the dominant rhetoric and even spill over into projects that are critical of the growth imperative and of platform-companies’ abuses of power and tendency to hide or dissimulate their carbon footprint (Caccamo, 2019; Durand-Folco, 2017).
However, the critical uses of digital technologies are not immune to the paradoxical dynamics of contemporary urbanization, whereby collective action tends to turn into maladaptation3 despite any other intentions (Schipper, 2020). As the climate crisis pushes problem-solving to its limits, the paradoxes of digital technology are essentially no more than an amplified reflection of a much deeper phenomenon.
2) What is a paradox?
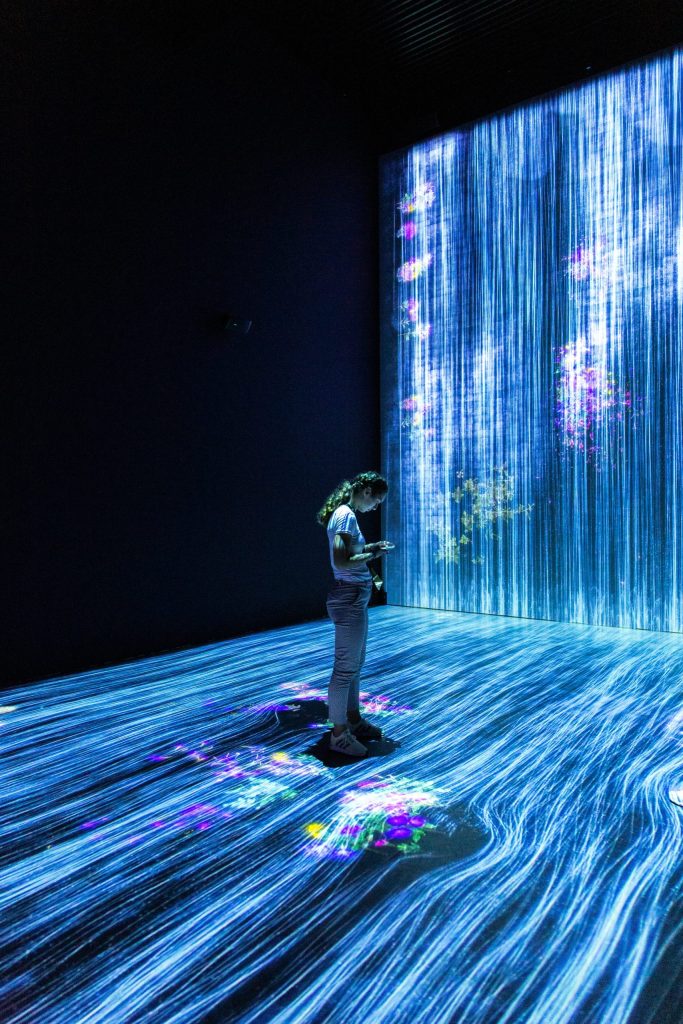
For the paradoxes referred to here, we use the definition “persistent contradictions between interdependent elements” (Schad et al., 2016, p. 10; own translation). The contradiction of a paradox is persistent in the sense that it resists any avenue of resolution; it is insoluble. This is why a paradox cannot be successfully resolved with the prevalent “problem-solving” approach, which defines our relationships to the world in all spheres of individual or collective action (family, professional, political, etc.). As any paradox is characterized by insolubility, those confronted with a paradox, be it an individual or a collective, will feel a violent sense of having reached an impasse; we think here of the growing number of young people who feel projected into the simultaneous role of saviour and victim in the face of the climate crisis (Benoit et al., 2022; Schwartz et al., 2022). The impasse becomes all the more paralyzing when the paradox that constitutes it remains implicit, or worse, is framed as a soluble problem.
So, rather than looking at the climate crisis in terms of problem-solving, our study pursues the idea that resilience in the face of the climate crisis cannot be conceived independently of the paradoxical logics that underpin it; hence, the paradox perspective.
3) The paradoxes of the smart city
In this study, we focus on two types of existential paradoxes that strike certain elected officials and municipal administrators: the paradox of the rebound effect, in connection with climate change, and the paradox of predatory emancipation, in connection with social inequities.
3.1 The climate emergency and the paradox of the rebound effect
Recently, the rebound effect has been the subject of growing interdisciplinary attention, and has even appeared in the popular media (see e.g., Bédard, Les Affaires, March 15, 2018; Harvey, Le Devoir, November 7, 2015). In the smart city, it typically corresponds to the paradoxical phenomenon whereby a technology, public policy or circular economy strategy designed to reduce the consumption of an energy resource turns against itself, provoking behaviours or systemic dynamics that cancel out some or all of the expected environmental gain (Font Vivanco et al., 2018). Take, for example, the rising use of machine learning in a manufacturing context, which enables gains in energy efficiency and thus reductions in greenhouse gas (GHG) emissions. If these efficiency gains were to translate into increased production, as might be expected, then the gains would be partially or totally offset. This means that GHG emissions could then be increased compared to the initial situation without AI. Without even taking account of the intrinsic carbon of AIs, we would then be faced with a direct rebound effect on the scale of a manufacturing company or an entire industrial sector.
When a policy is put in place to combat climate change, the potential gains are greater, but so is the scale of the rebound effect (Dauvergne, 2022). In terms of mobility, for example, the installation of a fleet of shared electric vehicles has the potential to improve energy efficiency and the carbon footprint. That said, the opposite could also hold true, for, if a critical mass of people were to substitute not their car but public transport with electric vehicles, GHG emissions would increase (Herrmann et al., 2014).
In addition, the modeling of rebound effects for the purposes of decision support or climate policy development poses major challenges. Typically, economic models are combined with environmental impact models such as life cycle assessment (Font Vivanco and van der Voet, 2014). With the advancement of complementary analytical methods (e.g., deep learning), hybridization continues, giving rise to models that are increasingly sophisticated (OECD, 2019) but also increasingly energy-intensive (Almeida et al., 2018; Wu et al., 2017). As a result, the causal chains to be understood are so extensive, uncertain and entangled that even the best models are unable to establish the necessary and sufficient conditions for avoiding a rebound (Zink and Geyer, 2017). It is as if the rise of digital environmental modelling has contributed to constantly broadening the scope of the phenomenon being modelled, thereby making it ever more elusive.
Whatever its scale, the rebound effect presents itself as a paradoxical dynamic that should not automatically be assimilated to the soluble-problem model. When it comes to urban climate action, the paradoxical perspective at least gives us the ability to recognize that the digital remedy is also the poison.
3.2 Personal data security and the paradox of predatory emancipation
It is against the backdrop of the climate emergency, alongside other issues pertaining to good urban governance (such as security or social equity), that sensors are multiplying in common-use urban spaces, both public and private. But while the smart-card type of usage associated with smartphones remains under the partial control of its users, the networks of sensors (cameras, pollution or noise sensors, etc.) weaving their way invisibly through the urban fabric mark the advent of more intrusive approaches. In the near future, for example, we can expect the Internet of Things to increase the number of sensors in urban areas as well as the number of interfaces that can exchange data more or less freely.
When it comes to the AI systems that enable the analysis of the data continuously emitted and captured by these networks of connected objects, the issues of social inequity and exclusion are numerous and abundantly documented. They are typically associated with the biases inscribed in the training data of learning algorithms, which are then introduced to AI-assisted decision-making processes. The phenomenon of predictive policing has drawn attention in recent years, with algorithms designed “to predict where and when crimes are likely to take place, but also and above all to guide, supervise and regulate police work” (Benbouzid, 2018, p. 223; own translation). However, so far they have tended to exacerbate inequities and violence, particularly toward racialized populations (Asaro, 2019; Niculescu-Dincă, 2018)—an issue that is not specific to predictive policing. All the already existing social biases are likely to be reinforced whenever an AI system assists in making a decision with a high social impact (Bender et al., 2021), for example, in the supposedly more objective allocation of healthcare services (Obermeyer et al., 2019). Moreover, in the name of good governance principles, such as safety, equity and inclusion, the multiplication of AIs could make it virtually impossible for individuals to travel without a digital trail, compromising not only privacy but also the very values of equity and inclusion this technology was intended to defend in the first place (Castets-Renard et al., 2020; CNIL, 2017).
It is in this sense that the urban uses of AI give rise to a paradox of predatory emancipation analogous to the paradox of the rebound effect. Whatever the intended purpose, the data that feed it always carry both an emancipatory potential and a risk of predatory practices. Here again, the paradoxical perspective does, if minimally, enable us to recognize that the digital remedy is also the poison.
Conclusion
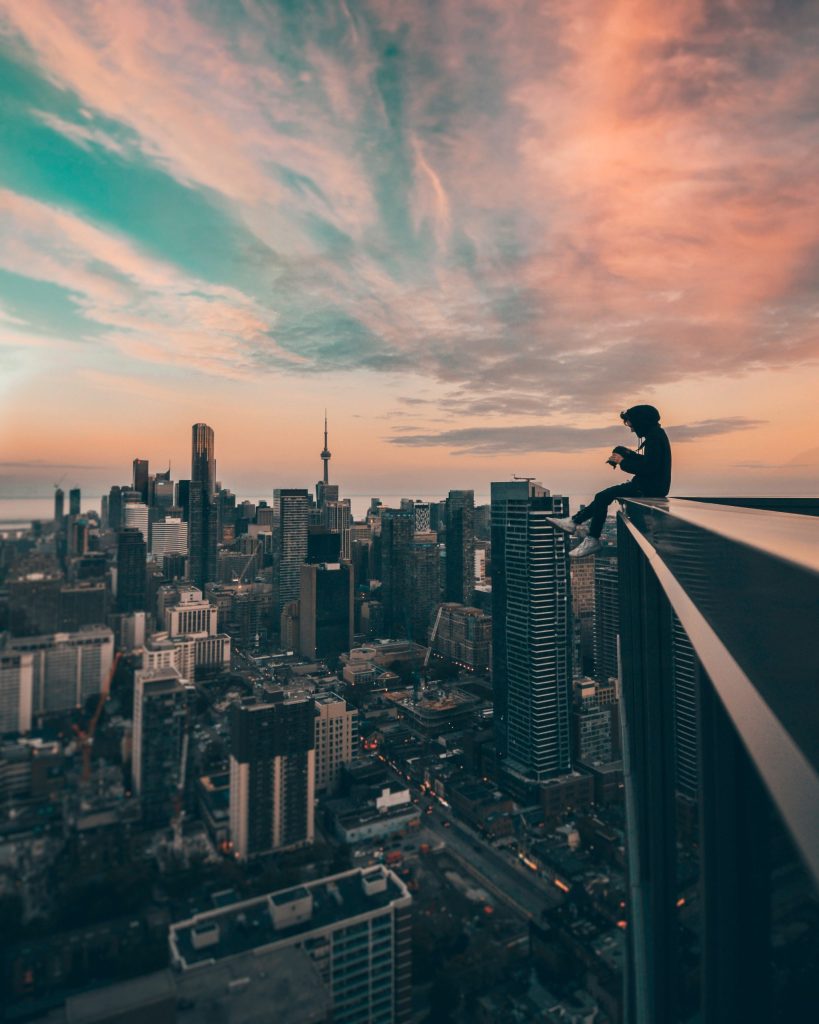
Overall, when applied to urban climate action, megadata flows and the AI systems that enable their analysis are subject to a paradox: they can equip people and communities with new capabilities to fight and adapt; however, they can also make them vulnerable in new ways. AI algorithms that reduce GHG emissions by improving the energy efficiency of a given system (e.g., MaaS (Mobility as a Service)) themselves require huge amounts of energy to train and deploy. Following the phenomenon of the rebound effect, the savings generated by these AIs on a given scale tend, moreover, to be transposed in the form of systemic dynamics on a larger scale, which reduce or cancel out the expected environmental benefit. Similarly, AI algorithms designed to combat social inequities tend to insidiously perpetuate these very inequities via the biases inscribed in the training data. In this sense, digital paradoxes are not problems to be solved: their dynamics reflect the limits of problem-solving to which we have collectively become blind.
However, the intensity of digital data flows at the root of this twofold paradox has given rise to a synergy of actions: by aiming for digital sobriety or, in other words, reducing the flow of data put into circulation to the strict minimum (to be defined according to the context), we are at the same time reducing in absolute terms the energy consumed and the GHGs emitted by digital technologies.
In practice, it is not all that simple, as the raison d’être of the emerging 5G technology is precisely to improve the energy efficiency of flows, and therefore drastically increase the data rate in circulation for the same amount of energy (Wu et al., 2017). It is worth noting, however, that the environmental benefits associated with digital sobriety translate perfectly to the social level via the principle of data minimization (EU, 2018). In other words, by collecting only the personal data that is necessary for the purpose for which it is being processed, and according to the “informational norms” specific to a particular social context (Nissenbaum, 2018), we give ourselves the means to generate a virtuous circle both environmentally and socially.
- Problem-solving is the “capacity [of an individual or collective] to consider the probable regarding the certain, the desperate regarding assurance, chance regarding conduct, and the harmful regarding perfection” (Vérin, 1984, p. 262; own translation). ↩︎
- The speech given in November 2008 by Samuel J. Palmisano, then CEO of IBM Corporation, fully embodied the trend of the moment, while heralding the discourse that has now become dominant. ↩︎
- E. Lisa F. Schipper (2020), author and member of Working Group II (WG2) of the 6th IPCC Assessment Report, defines maladaptation as follows: “Maladaptation is not just a waste of time and money; it is when an action results in conditions that are worse than those which the original strategies were trying to address. Maladaptation is therefore not being poorly adapted to climate change; it is actually a process whereby people become even more likely to be negatively affected by climate change.” (p. 409) ↩︎
To cite this article
Arpin, M-L., Gambs, S. (2022). Digital technologies to combat climate change: Between problem and paradox. In Cities, Climate and Inequalities Collection. VRM – Villes Régions Monde. https://www.vrm.ca/les-technologies-numeriques-pour-lutter-contre-les-changements-climatiques-entre-probleme-et-paradoxe-2
Reference Text
Arpin, M.-L., et Gambs, S. (2022). Synthèse des états de l’art sur les enjeux éthiques de la ville intelligente : une perspective des paradoxes. Rapport préparé dans le cadre du programme Innovation du ministère de l’Économie et de l’Innovation (MÉI) du Québec
References
Aïvodji, U., Arai, H., Fortineau, O., Gambs, S., Hara, S., et Tapp, A. (2019). « Fairwashing: The Risk of Rationalization ». Dans International Conference on Machine Learning (p. 161‑170).
Albino, V., Berardi, U., et Dangelico, R. M. (2015). « Smart Cities: Definitions, Dimensions, Performance, and Initiatives ». Journal of Urban Technology, 22(1), p. 3‑21.
Almeida, F., Assunção, M. D., Barbosa, J., Blanco, V., Brandic, I., Da Costa, G., Dolz, M. F., Elster, A. C., Jarus, M., Karatza, H. D., Lefèvre, L., Mavridis, I., Oleksiak, A., Orgerie, A.-C., et Pierson, J.-M. (2018). « Energy Monitoring as an Essential Building Block towards Sustainable Ultrascale Systems ». Sustainable Computing: Informatics and Systèmes, 17, p. 27‑42.
Arpin, M.-L. (2020). L’ingénieur : entre problème et paradoxe [thèse de doctorat, Université du Québec à Montréal, Canada]. Archipel.
Arrieta, A. B., Díaz-Rodríguez, N., Del Ser, J., Bennetot, A., Tabik, S., Barbado, A., … & Herrera, F. (2020). Explainable Artificial Intelligence (XAI): Concepts, taxonomies, opportunities and challenges toward responsible AI. Information Fusion, 58, 82-115.
Asaro, P. M. (2019). « AI Ethics in Predictive Policing: From Models of Threat To an Ethics of Care ». IEEE Technology and Society Magazine, 38(2), p. 40‑53.
Bakis, H., et Dupuy, G. (2012). « À propos de la notion de “villes intelligentes””. Netcom. Réseaux, communication et territoires, 26(3/4), p. 373‑374.
Barnett, C., et Parnell, S. (2016). « Ideas, Implementation and Indicators: Epistemologies of the Post-2015 Urban Agenda ». Environment and Urbanization, 28(1), p. 87‑98.
Beck, U. (2008). La société du risque : sur la voie d’une autre modernité. Flammarion.
Bédard, Les Affaires, 15 mars 2018
Benbouzid, B. (2018). « Quand prédire, c’est gérer. La police prédictive aux États-Unis ». Réseaux, 211(5), p. 221‑256.
Bender, E. M., Gebru, T., McMillan-Major, A., & Shmitchell, S. (2021, mars). « On the Dangers of Stochastic Parrots: Can Language Models Be Too Big? ». Dans Proceedings of the 2021 ACM Conference on Fairness, Accountability, and Transparency (p. 610‑623).
Benoit, L., Thomas, I., et Martin, A. (2022). « Review: Ecological Awareness, Anxiety, and Actions among Youth and their Parents – A Qualitative Study of Newspaper Narratives ». Child and Adolescent Mental Health, 27(1), p. 47‑58.
Caccamo, E. (2019). Critique de la « numérisation » croissante de la société: vers une trajectoire alternative et décroissante. In E. Caccamo, J. Walzberg, T. Reigeluth, & N. Merveille (Eds.), De la ville intelligente à la ville intelligible (1st ed., pp. 117–138). Presses de l’Université du Québec.
Caprotti, F., Cowley, R., Datta, A., Broto, V. C., Gao, E., Georgeson, L., Herrick, C., Odendaal, N., et Joss, S. (2017). « The New Urban Agenda: Key Opportunities and Challenges for Policy and Practice ». Urban Research & Practice, 10(3), p. 367‑378.
Carabantes, M. (2019). « Black-Box Artificial Intelligence: An Epistemological and Critical Analysis ». AI & SOCIETY, 35, p. 309‑317.
Castets-Renard, C., Guiraud, É., et Avril-Gagnon, J. (2020). « Cadre juridique applicable à l’utilisation de la reconnaissance faciale par les forces de police dans l’espace public au Québec et au Canada : éléments de comparaison avec les États-Unis et l’Europe ». Observatoire international sur les impacts sociétaux de l’IA et du numérique (OBVIA).
CEST. (2017). La ville intelligente au service du bien commun : lignes directrices pour allier l’éthique au numérique dans les municipalités au Québec [avis de la Commission de l’éthique en science et en technologie (CEST)]. Gouvernement du Québec.
CEST. (2020). L’Internet des objets, la vie privée et la surveillance : balises éthiques et recommandations [supplément d’avis de la Commission de l’éthique en science et en technologie (CEST)]. Gouvernement du Québec.
Clos, J. (2017). « Introduction », dans ONU-Habitat, 2016. Urbanization and Development: Emerging Futures. The World Cities Report 2016 (full version). Kenya : ONU-Habitat, p. iv‑v.
CNIL. (2017). La plateforme d’une ville : les données personnelles au cœur de la fabrique de la smart city. Cahiers IP (Innovation & Prospective) N° 05, Commission nationale de l’informatique et des libertés.
Dauvergne, P. (2022). « Is Artificial Intelligence Greening Global Supply Chains? Exposing the Political Economy of Environmental Costs ». Review of International Political Economy, 29(3), p. 696‑718.
De Certeau, M. (1990). L’invention du quotidien I : Art de faire. Gallimard.
Durand Folco, J. (2017). À nous la ville! : traité de municipalisme. Écosociété.
Floridi, L. (2019). « Translating Principles into Practices of Digital Ethics: Five Risks of Being Unethical ». Philosophy & Technology, 32(2), p. 185‑193.
Font Vivanco, D., et al. (2018). « Roadmap to Rebound: How to Address Rebound Effects from Resource Efficiency Policy. » Sustainability 10(6): 2009.
Font Vivanco, D. and E. van der Voet (2014). « The rebound effect through industrial ecology’s eyes: a review of LCA-based studies. » The International Journal of Life Cycle Assessment 19(12): 1933-1947.
Green, B. (2019). The Smart Enough City: Putting Technology in Its Place To Reclaim Our Urban Future. MIT Press.
Guldi, J. (2016). « A History of the Participatory Map ». Public Culture, 29(1), p. 79‑112.
Guldi, J. (2018). « Global Questions About Rent and the Longue Durée of Urban Power, 1848 to the Present ». New Global Studies, 12(1), p. 37‑63.
Halpern, O., LeCavalier, J., Calvillo, N., et Pietsch, W. (2013). « Test-Bed Urbanism ». Public Culture, 25(2), p. 272‑306.
Halpern, O. (2015). Beautiful Data. Duke University Press.
Halpern, O., Mitchell, R., et Geoghegan, B. D. (2017). « The Smartness Mandate: Notes toward a Critique ». Grey Room, 68, p. 106‑129.
Harvey, R. (7 novembre 2015). « Une approche globale pour réduire les GES. » Le Devoir.
Hatuka, T., Toch, E., Birnhack, M., et Zur, H. (2020). The Digital City: Critical Dimensions in Implementing the Smart City. SSRN.
Hatzivasilis, G., Christodoulakis, N., Tzagkarakis, C., Ioannidis, S., Demetriou, G., Fysarakis, K., et Panayiotou, M. (2019). « The CE-IoT Framework for Green ICT Organizations ». IEEE DCOSS, p. 29‑31.
Herrmann, S., Schulte, F., et Voß, S. (2014). « Increasing Acceptance of Free-Floating Car Sharing Systems Using Smart Relocation Strategies: A Survey Based Study of car2go Hamburg ». Computational Logistics, p. 151‑162.
Hollands, R. G. (2008). « Will the Real Smart City Please Stand Up? Intelligent, progressive or entrepreneurial? ». City, 12(3), p. 303‑320.
IPCC, (2022). Climate Change 2022: Impacts, Adaptation, and Vulnerability. Contribution of Working Group II to the Sixth Assessment Report of the Intergovernmental Panel on Climate Change [H.-O. Pörtner, D.C. Roberts, M. Tignor, E.S. Poloczanska, K. Mintenbeck, A. Alegría, M. Craig, S. Langsdorf, S. Löschke, V. Möller, A. Okem, B. Rama (eds.)]. Cambridge University Press.
Kaack, L. H., Donti, P. L., Strubell, E., Kamiya, G. Creutzig, F. et Rolnick, D. (2022). « Aligning Artificial Intelligence with Climate Change Mitigation ». Nature Climate Change, 12(6), p. 518‑527.
Kitchin, R. (2016). « The Ethics of Smart Cities and Urban Science ». Philosophical Transactions of the Royal Society A: Mathematical, Physical and Engineering Sciences, 374(2083).
Kitchin, R. (2014). « The Real-Time City? Big Data and Smart Urbanism ». GeoJournal, 79(1), p. 1‑14.
Leclerc, S. H., et Badami, M. G. (2020). « Extended Producer Responsibility for E-waste Management: Policy Drivers and Challenges ». Journal of Cleaner Production.
Malherbe, J.-F. (2000). Le nomade polyglotte. Bellarmin.
Mytton, D. (2020). « Hiding Greenhouse Gas Emissions in the Cloud ». Nature Climate Change, 10(8), p. 701.
Niculescu-Dincă, V. (2018). « Towards a Sedimentology of Information Infrastructures: A Geological Approach for Understanding the City ». Philosophy & Technology, 31(3), p. 455‑472.
Nissenbaum, H. (2018). « Respecting Context to Protect Privacy: Why Meaning Matters ». Science and Engineering Ethics, 24(3), p. 831‑852.
Obermeyer, Z., Powers, B., Vogeli, C., Mullainathan, S.(2019). « Dissecting Racial Bias in an Algorithm Used to Manage the Health of Populations ». Science, 366(6464), p. 447‑453.
Palm, E., et Nikoleris, A. (2021). « Conflicting Expectations on Carbon Dioxide Utilisation ». Technology Analysis & Strategic Management, 33(2), p. 217‑228.
Picon, A. (2015). Smart Cities: A Spatialised Intelligence. Wiley.
Picon, A. (2016). « L’avènement de la ville intelligente ». Sociétés, 132, p. 9‑24.
Revi, A., et al. (2014). « Towards transformative adaptation in cities: the IPCC’s Fifth Assessment. » Environment and Urbanization 26(1): 11-28.
Schad, J., Lewis, M. W., Raisch, S., et Smith, W. K. (2016). « Paradox Research in Management Science: Looking Back to Move Forward ». Academy of Management Annals, 10(1), p. 5‑64.
Schipper, E. L. F. (2020). « Maladaptation: When Adaptation to Climate Change Goes Very Wrong ». One Earth, 3(4), p. 409‑414.
Schwartz, S. E. O., Benoit, L., Clayton, S., Parnes, M. F., Swenson, L., et Lowe, S. R. (2022). « Climate Change Anxiety and Mental Health: Environmental Activism as Buffer ». Current Psychology.
Taddeo, M., et Floridi, L. (2018). « How AI Can Be a Force for Good ». Science, 361(6404), p. 751‑752.
Truby, J. (2020). « Governing Artificial Intelligence to Benefit the UN Sustainable Development Goals ». Sustainable Development, 28(4), p. 946‑959.
Union européenne. (2018). Règlement général sur la protection des données (RGPD) de l’Union européenne. Journal officiel de l’Union européenne.
UN-Habitat. (2016). Urbanization and Development: Emerging Futures. The World Cities Report 2016 (full version). Kenya : UN-Habitat.
UN. (1976). « Déclaration de Vancouver sur les établissements humains ». Dans le rapport d’habitat : Conférence des Nations Unies sur les établissements humains (Habitat I) à Vancouver, du 31 mai au 11 juin 1976. Organisation des Nations Unies, Vancouver, Canada.
Vérin, H. (1984). Entrepreneurs, entreprise : histoire d’une idée. Classiques Garnier.
Vérin, H. (1993). La gloire des ingénieurs : l’intelligence technique du XVIe au XVIIIe siècle. Albin Michel.
Vinuesa, R., Azizpour, H., Leite, I., Balaam, M., Dignum, V., Domisch, S., Felländer, A., Langhans, S. D., Tegmark, M., et Nerini, F. F. (2020). « The Role of Artificial Intelligence in Achieving the Sustainable Development Goals ». Nature Communications, 11(233), p. 1‑10.
Wu, Q., Li, G. Y., Chen, W., Ng, D. W. K., et Schober, R. (2017). « An Overview of Sustainable Green 5G Networks ». IEEE Wireless Communications, 24(4), p. 72‑80.
Zink, T., et Geyer, R. (2017). « Circular Economy Rebound ». Journal of Industrial Ecology, 21(3), p. 593‑602.